CHALLENGE
A global pharmaceutical leader was in the process of reinventing its drug development process to become faster and more efficient. One of the initiatives they were looking at was inSilico drug development – a data-driven approach to drug development that aims to maximize the use of data and data models to speed up the decision-making in the drug development process and shorten the whole process.
Their pharma research team was looking for a partner who could help them with advice on data and data science topics and at the same time support the business transformation process.
SOLUTION
Using data science a multidisciplinary team of pharma scientists, data scientists, and business consultants was formed that led the initiative from its first step, the feasibility study, to a full scope implementation and roll out in multiple locations around the world.
One of the main deliverables of the project was the inSilico process – a new aspect of the drug development process that helps pharma scientists to:
Acquire and validate drug development data quickly
Analyze R&D data using best-practice analysis concepts and tools
Simulate drug behavior using data-driven models
The final product of this process is a pharma digital twin – a model that engrains most of the experience gained in the development process and can be used during the product life cycle to
Troubleshoot production issues
Simulate the impact of changes of ingredients or technological processes
Optimize production site transfers
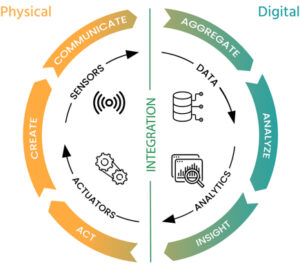
BENEFITS
Faster and more informed day-to-day decision making by pharmaceutical R&D teams
Standardized analytical tools allow team members to gain more insights
inVitro and inVivo models allow omission of certain physical experiments contributing to less effort and cost reduction
The inSilico process that links all the activities (data – analysis – modeling – inSilico experiments) enables the customer to speed up the drug development process and reduce costs by a significant factor.
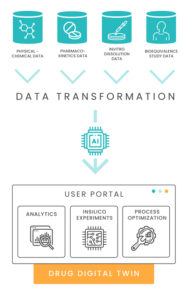